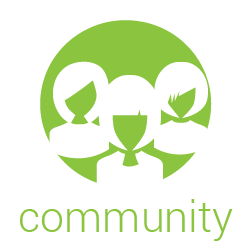
Salon E
Detecting Gradients in the Microbiome Data Using Indirect Ordination Methods (303712)
*Lan Huong Nguyen, Stanford Institute for Computational & Mathematical EngineeringOrdination tools are frequently used for data exploration in the microbiome research. The use of the ordination methods rarely goes beyond PCoA plots, and a qualitative assessment of the trends discovered. Here we present a systematic procedure for studying the gradients. We show how to best estimate the gradients, how to interpret the gradient curves in terms of associated environmental variables, and how to remove the strongest gradient in order to study the remaining variability in the abundance data. A number of ordination techniques were tested on their ability to detect gradients in both simulated and real microbial data. We found that in general, a lot of the distance based ordination methods work best when applied to the presence/absence instead of the count data. Two effective methods for inferring the samples ordering along the estimated gradient are presented; one is based on constructing a minimum spanning tree and the other on a computing principal curve. The ordering can be used to evaluate the importance of the covariates on the gradient in the abundance data.