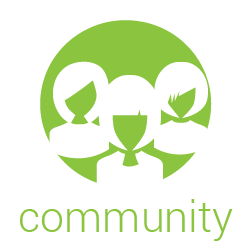
Salon 3
Statistical Fusion Learning: Combining Inferences from Multiple Sources for More Powerful Findings (303557)
*Regina Y Liu, Rutgers UniversityKeywords: Confidence distribution, data depth, fusion learning, meta-analysis
Inferences from multiple databases or studies can often be fused together to yield a more powerful overall inference than individual studies alone. Fusion learning refers to the development of such effective approaches to synergize learnings from different sources. Effective fusion learning is particularly important in this era of data explosion, with troves of data nowadays collected routinely from diverse sources in all domains and at all time.
Using the tracking of aircraft landing performance as an illustrative example, we present a powerful fusion learning approach. Specifically, we apply the concepts of confidence distribution (CD) and data depth to develop a new nonparametric approach for combining inferences from multiple studies for a common hypothesis. We discuss several new approaches in fusion learning in the context of combining test results from independent studies or joint modeling of data from possibly heterogeneous sources. These approaches are completely data driven and have several desirable properties. Examples of simulation data and aircraft landing data will be presented.