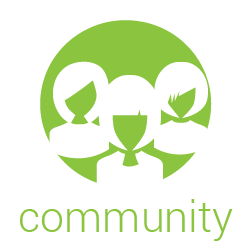
Salon 3
Cluster Analysis of Longitudinal Profiles with Subgroups (303513)
Annie Qu, University of Illinois at Urbana-Champaign*Xiaolu Zhu, University of Illinois at Urbana-Champaign
Keywords: ADMM, longitudinal data, minimax concave penalty, model selection, nonparametric
In this talk, we propose a penalized regression method to cluster profiles of longitudinal data. The novelty of our approach is that we allow longitudinal patterns from each subject to be unique, which can be modeled through nonparametric approaches such as the B-spline method. We identify clusters by applying a nonparametric pairwise grouping penalization to their associated parameters, and therefore distinguish clusters based on different patterns of predicted longitudinal curves. One advantage of our proposed method is that we do not need to pre-specify the number of clusters; instead it is selected automatically through a model selection criterion. Our method is also applicable for unbalanced data where different subjects could have different time points of measurements. To implement our proposed method, we develop an alternating direction method of multipliers (ADMM) algorithm which has the convergence property. In addition, we establish theoretical properties for consistency, and illustrate that our method outperforms existing competitive approaches in our numerical studies.