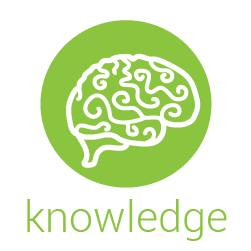
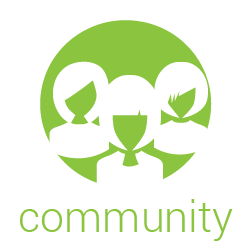
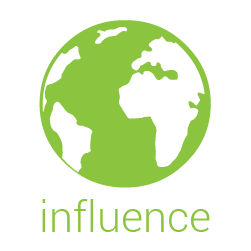
Carolina Ballroom
Approximate Confidence Distribution (ACC) Computing Method (303512)
*Suzanne Thornton, Rutgers UniversityApproximate Bayesian computation (ABC) methods have grown increasingly popular since early applications in population genetics. However, the theoretical justification for inference based on such methods has yet to be fully developed, especially for cases in which the summary statistic used in the ABC algorithm is not sufficient. By taking advantage of the development on confidence distributions, we consider a more general computational technique called approximate confidence-distribution computation (ACC). The ACC method extends the traditional ABC framework to a frequentist setting where no prior assumptions are necessary. When the choice of proposal function in ACC coincides with the prior function in ABC, the two methods are the same. ACC however, can be applied more broadly since the choice of the proposal function is not limited to the prior. Unlike the ABC approach, we can ensure the correct frequentist coverage property necessary to justify conducting inference, whether the summary statistic used in the algorithm is sufficient or not. This work offers a more extensive alternative to a popular likelihood-free Bayesian method.