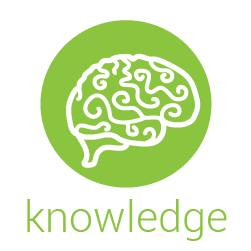
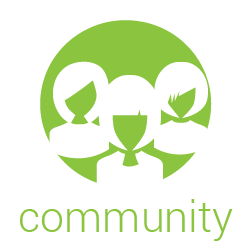
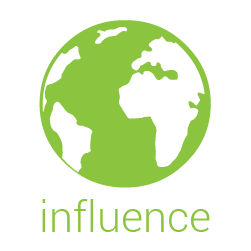
Carolina Ballroom
Generalized Extreme Value Regression for Binary Response Data: A Latent Factor Model for Spatial Data with Non-Random Missingness (303511)
*Xiaoyue Zhao, University of MinnesotaUsually a large amount of data is collected during a periodontal exam. There are challenges when analyzing these data. We cannot apply the traditional generalized liner mixed (GLM) model to these spatially-referenced data with a skewed binary response. Most patients have missing teeth and the number and location of missing teeth inform about the patient’s periodontal health. This paper presents a flexible link function for modeling skewed binary response data with individual level and spatial covariates as a function of a multivariate latent variable through the mean based on the generalized extreme value (GEV) distribution. The missingness is jointly modeled using the same multivariate latent variable. The parameters are estimated by using the Metropolis-Hastings algorithm since there’s no closed form for the full conditionals associated with the GEV distribution. We show using simulated and real data that incorporating spatial association and joint-modeling the binary response and missingess location mitigates the problems presented by the data.