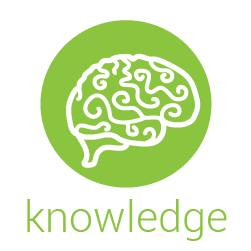
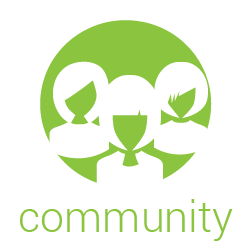
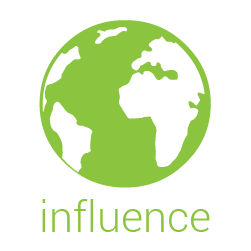
Carolina Ballroom
Generalized R-Squared for Detecting Non-Independence (303510)
*Xufei Wang, Harvard UniversityDetecting whether two random variables are non-independent is a fundamental problem in statistics and machine learning. Although the celebrated Pearson correlation is effective for capturing linear dependency, it can be totally powerless for detecting nonlinear and heteroscedastic patterns. To cope with these shortcomings, we introduce a new statistics, G-squared, as a generalization of the classic R-squared to test whether two univariate random variables are mutually independent and to measure the strength of their relationship. The G-squared is almost identical to the R-squared if the underlying relationship is indeed linear, and is powerful at capturing nonlinear relationships effectively. Another attractive feature is that the G-squared has an intuitive meaning of the piece-wise R-squared between the two variables. Through an intensive simulation study, we observed that the G-squared is always one of the most powerful performers among some state-of-art methods.