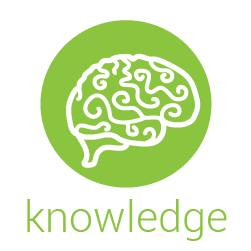
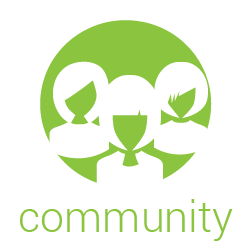
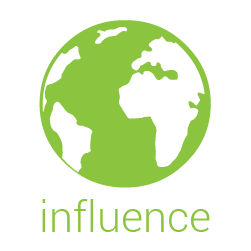
Carolina Ballroom
Differentially Private Data Synthesis Partitioning for Big Data (303507)
*Claire McKay Bowen, University of Notre DameAs the era of information and technology continues to dominate, big data offers tremendous benefits for education, economics, medical research, national security, and other areas through data-driven decision-making, insight discovery, and process optimization. However, one of the significant challenges in analyzing big data is the extreme risk of exposing personal information of individuals who contribute to the data when sharing it among collaborators or releasing it publically. An intruder could identify a participant by isolating the numerous connections to other contributors within the big data set. One method that preserves differentially privacy, a condition on data releasing algorithms that quantifies disclosure risk, is model-based differentially private data synthesis (modips). While guaranteeing privacy at a prespecified level, this technique perturbs the parameters of interest, and then generates the synthetic data through multiple synthesis from predicted values in a Bayesian framework. We present various partitioning approaches using modips on big data sets to improve the statistical utility and compare them to provide guidance on practical feasibility.