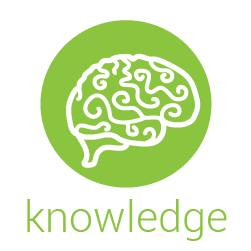
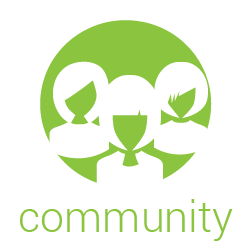
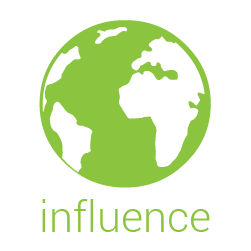
Carolina Ballroom
B2M2 Tree: A Decision Tree Method for Modeling Correlated Binary Outcomes (303496)
*Jaime Lynn Speiser, Medical University of South CarolinaCorrelated binary outcomes are frequently encountered in clinical research. Generalized linear mixed models (GLMMs) typically employed for correlated variables require assumptions which are inappropriate for some datasets. We develop an alternative method with fewer assumptions called Bayesian Binary Mixed Model (B2M2) trees, which combines decision trees and Bayesian GLMMs. Simulation studies show that B2M2 trees offer similar or superior accuracy compared to standard classification and regression trees and Bayesian GLMMs for correlated binary outcomes. The method is applied to a real dataset from the Acute Liver Failure Study Group to predict daily outcome of poor or favorable condition using clinical variables. B2M2 trees offer an alternative method for modeling binary outcomes which may be applied in myriad research settings when data are repeatedly collected.