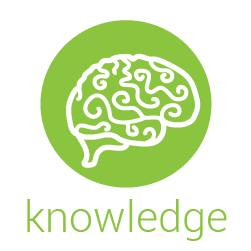
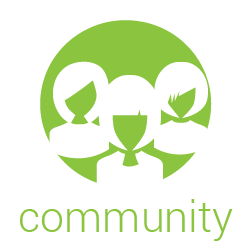
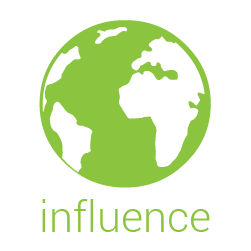
Carolina Ballroom
Sponsored by Bank of America
WITHDRAWN: Sufficient Dimension Reduction for Big Data Analysis (303431)
*Nusrat Jahan, James Madison UniversityMachine learning is a technique- widely used in big data analysis. This is an iterative process of model building for multifaceted data structure. This approach allows model building based on the data (training data), models are independently adaptable when exposed to new data (test data). Unfortunately this approach could produce hundreds of models, a large portion of those would be spurious related to a specific target. The concept of sufficient dimension reduction (SDR) was formally introduced by Dennis Cook in 1994. SDR is a process of extracting key information by removing uninformative variance from a data set. A sparse sufficient reduction can be applied on the selected set of predictors to obtain a linear combination of the most important sets of predictors. In this work we propose a sparse sufficient dimension reduction technique to identify linear combinations of the most important input variables that best explain the target variable prior to machine learning model building.