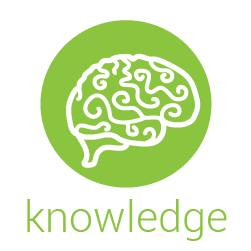
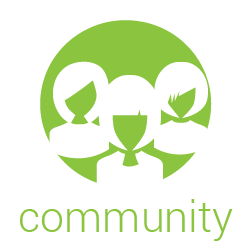
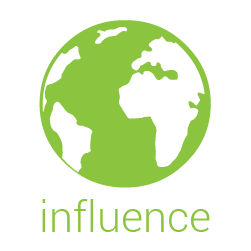
Carolina Ballroom
Sponsored by Bank of America
Model Selection Probabilities (303420)
*Xin Lu Tan, The Wharton School"We consider the estimation of true model/variable selection probabilities in the context of regression. We show, through a simple slope test example, that bootstrap fails in estimating model selection probabilities. Indeed, we establish a rigorous impossibility result that no method is able to consistently estimate model selection probabilities for data of size n from a dataset of the same size. We then show that the m-out-of-n bootstrap can consistently estimate selection probabilities for data of size m = o(n) based on a sample size n. We establish the asymptotic normality of the m-out-of-n bootstrap estimator, allowing m to grow with n subject to m = o(n) and provide a consistent estimator for its asymptotic variance. This leads to asymptotically valid confidence intervals for selection probabilities associated with data of size m. We examine how true model selection probabilities change with sample sizes for several popular model selection methods on simulated data examples. Some of these examples illustrate the impossibility of extrapolating from small values of m to the actual sample size n, which agrees with our impossibility result. "