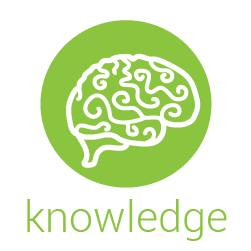
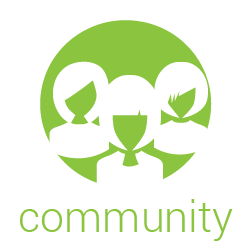
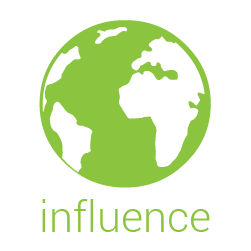
Carolina Ballroom
Sponsored by Bank of America
Robust Adaptive Group-Lasso for Functional Regression Model (303419)
*Jasdeep Pannu, Lamar UniversityFunctional data is becoming common these days. The presence of outliers adds extra complication to the estimation of the functional coefficients. The objective of this research is to develop a robust functional variable selection method for a functional regression model with a scalar response and functional predictors in the presence of outliers. Recently, a method called functional LAD-groupLASSO (LAD-gLASSO) has been developed which using the combination of the well-known robust function least absolute deviation (LAD) and the penalty function group LASSO minimizes the sum of the absolute value of the errors and penalizes the parameter functions. However, in this method same amount of penalty is applied to all the parameters. In order to reflect some reflect some subjectivity about the true parameter functions and to allow for different shrinkage and smoothness for the different functional predictors, we propose an alternative penalty function based on adaptive weights for the penalized estimation criterion. This method is called LAD-Adaptive group LASSO (LAD-agLASSO). In order to show the performance of the proposed method, we conduct an extensive simulation and use a real data.