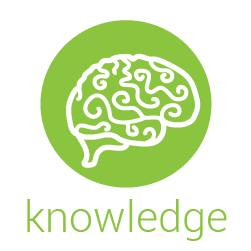
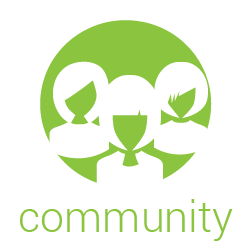
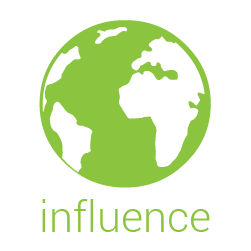
Carolina Ballroom
Sponsored by Bank of America
Multivariate Pattern Analysis and Confounding in Neuroimaging (303413)
*Kristin A Linn, University of PennsylvaniaNeuroimaging studies often quantify disease-related structural brain differences between populations using a multivariate pattern analysis (MVPA) such as the support vector machine (SVM). The SVM is trained to discriminate between groups, and the weights indicate which brain regions jointly drive the discriminative rule. However, classifier training in the presence of confounders may lead to identification of false disease patterns and spurious results. This occurs when classifiers rely heavily on regions that are strongly correlated with the confounders instead of regions that encode subtle disease changes. The imaging literature recommends using parametric models to regress out confounder effects at each brain region before SVM training. We show that this approach does not properly address the issue of confounding in MVPA. Instead, we propose a novel method that incorporates inverse probability weighting (IPW) during classifier training.