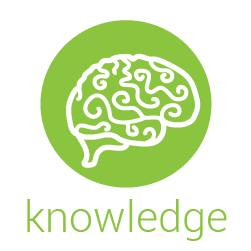
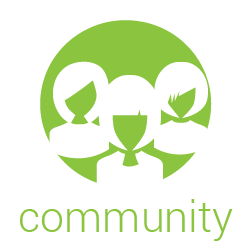
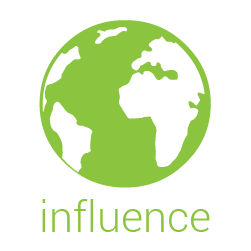
Carolina Ballroom
Sponsored by Bank of America
The Role of Penalized Regression in Clinical Research: When Should it be Used? (303412)
*Anne Eaton, University of MinnesotaIt’s well-known that stepwise variable selection methods can lead to biased coefficients and overfit models. However, clinical applications where paring down the number of variables falls to the statistician are ubiquitous and thus data-driven techniques are needed. Penalized regression methods (including LASSO, ridge and elastic net) have been heralded as a solution and are easily implemented in R and SAS. Because the limitations of these methods are not well understood, one may get the impression that they could be used in any and all model building scenarios. We used simulations to understand when they work well (and when they don’t). Penalized methods optimize prediction and do not produce unbiased coefficient estimates; as expected we observed consistent severe underestimation of model coefficients. This lead to predicted probabilities with a small spread and calibration slopes less than one. In small datasets, penalized methods often failed to select the correct model. The performance improves as sample size increases and we outline, under various scenarios and modeling goals, what sample size is needed to feel comfortable that using these methods are appropriate.