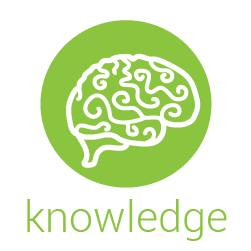
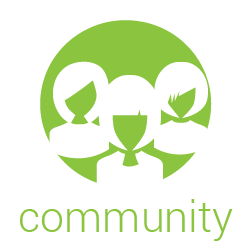
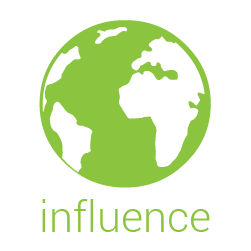
Carolina Ballroom
Sponsored by Bank of America
PCA Leverage: Outlier Detection for High-Dimensional fMRI Data (303411)
*Amanda Mejia, Johns Hopkins UniversityOutlier detection for high-dimensional data is a popular topic in modern statistical research. However, one source of high-dimensional data that has received relatively little attention is functional magnetic resonance images (fMRI), which consists of 100,000 or more measurements, sampled at hundreds to thousands of time points. At a time when the availability of fMRI data is rapidly growing, automated quality control and outlier detection methods are greatly needed. I propose PCA leverage and demonstrate how it can be used to identify outlying time points in an fMRI scan. I also propose an alternative measure, PCA robust distance, which is less sensitive to outliers and has controllable statistical properties. The proposed methods are validated through simulation studies and are shown to have high sensitivity and specificity. A reliability study using resting-state fMRI data shows that removal of outliers using the proposed methods results in more reliable estimation of subject-level resting-state networks using ICA.