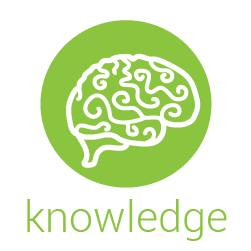
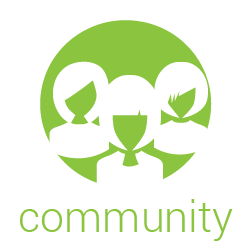
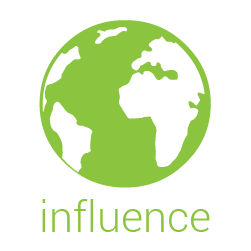
Carolina Ballroom
Sponsored by Bank of America
Lagged Kernel Machine Regression for Identifying Time Windows of Susceptibility to Complex Metal Mixture Exposures (303407)
*Shelley Han Liu, Harvard UniversityA critical public health concern is the impact of neurotoxic chemicals on children’s health; exposures to metal mixtures during early life may impact cognitive function, and there may exist critical time intervals during which vulnerability is increased. However, there is a lack of statistical methods to study time-varying exposures of complex toxicant mixtures. Therefore, we develop a flexible statistical method, Lagged Kernel Machine Regression (LKMR), to identify critical exposure windows of chemical mixtures that accounts for complex non-linear and non-additive effects of the mixture at any given exposure window. LKMR is a Bayesian hierarchical model that estimates how the effects of mixture exposures change with the exposure window using a novel grouped, fused Lasso for Bayesian shrinkage. Simulation studies demonstrate the performance of LKMR under realistic exposure-response scenarios, and demonstrate large gains over approaches that consider each critical window separately, particularly when serial correlation among the time-varying exposures is high.