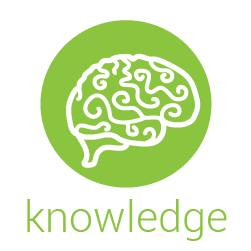
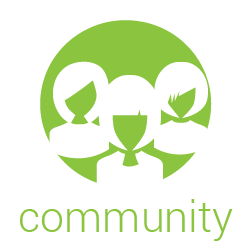
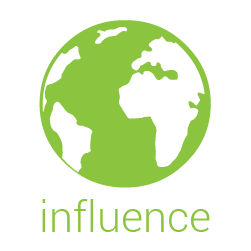
Carolina Ballroom
Sponsored by Bank of America
A Bayesian Framework for Identifying Placebo Responders in Clinical Trials (303406)
*Reagan M Rose, Harvard UniversityThe placebo serves as an indispensable control in randomized clinical trials and allows us to draw causal conclusions about the effect of drugs. However, in trials with a subjective primary outcome where the expected placebo effect is large, the placebo can become more of a confounder than a control. We develop a Bayesian framework for analysis of the placebo response using the Neyman-Rubin model of causal inference, which can be applied post-hoc to clinical trial data in order to obtain better estimates of the drug effect. We propose that all units in a clinical trial can be classified as either placebo “responders” or “non-responders” and that the observed outcomes for each unit can be viewed as manifestations of an underlying distribution that is dependent on this missing “responder” status. Viewing the marginal distribution of outcomes as a finite mixture model, we then implement missing data methods via MCMC in order to generate posterior probabilities that each unit is a placebo “responder” given their observed outcome. This general framework is extended to incorporate pre-treatment covariates, allowing more flexible modeling.