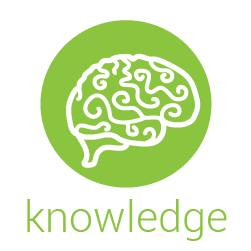
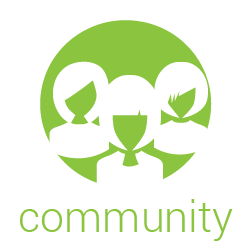
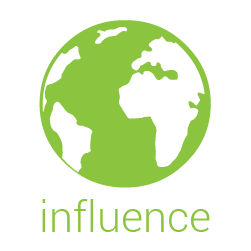
Carolina Ballroom
Sponsored by Bank of America
A Bayesian Hierarchical Model for Discrete Choice Data in Health Care (303399)
*Anna Liza Malazarte Antonio, University of California, Los AngelesDiscrete choice data provide health care researchers with an opportunity to study individual-specific patient preferences; however, these data are often sparse, due to the limited number of choice sets presented to each patient, and may contain missing ranking information. Moreover, methods to identify outliers in discrete choice data are lacking. We develop a Bayesian hierarchical random effects rank-ordered multinomial logit model for discrete choice data. Missing ranks are accounted for by marginalizing over all possible permutations of unranked alternatives to estimate individual-specific regression coefficients, modeled as a function of the individual-specific covariates. We provide a Bayesian version of measures of relative attribute importance with standard deviations. We propose modifications of the conditional predictive ordinate to identify individuals with outlying preferences with respect to the population and outlying choice sets that deviate from estimated individual-specific preferences. We apply the model to data from a study using a discrete choice experiment to estimate individual-specific preferences for health states related to prostate cancer treatment.