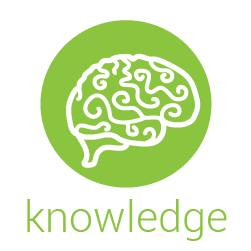
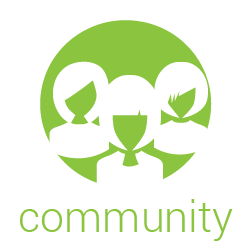
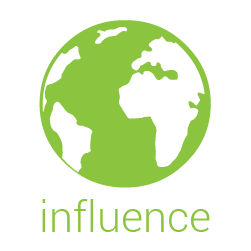
Salon 2
Parameter Inference for Coupled Stochastic Differential Equations (303278)
Harish Bhat, University of California, Merced*R. W. M. A Madushani, University of California, Merced
Keywords: Stochastic differential equations, density tracking, parameter inference, maximum likelihood estimation
We consider the problem of modeling vector-valued time series data using coupled stochastic differential equations. A key challenge in developing practical inference algorithms is the computation of both the likelihood and its gradient with respect to model parameters. We address these challenges through a novel and fast method to track the transition densities of the stochastic differential equation. The method applies quadrature to the Chapman-Kolmogorov equation associated with a temporal discretization of the stochastic differential equation. We use an adjoint base approach to compute the gradient of the likelihood in an efficient way as oppose to the direct sensitivity approach. Our method can be used to solve large-scale inference problems with a large number of unknown parameters by using quasi-Newton optimization algorithms to minimize the negative log likelihood function.