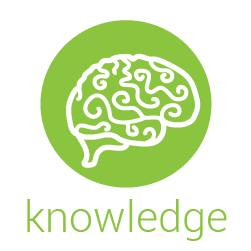
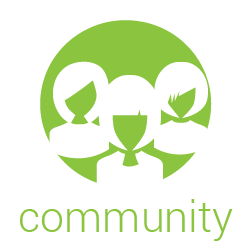
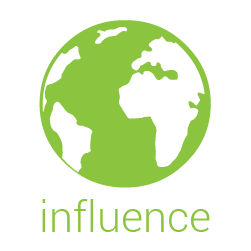
Salon 2
Using observed outcomes to design high-dimensional propensity scores (303257)
*Lo-Hua Yuan, Harvard UniversityKeywords: propensity score variable selection, high-dimensional propensity score, prognostic variables, split-data, cross-validation
Propensity score (PS) methods form the backbone of many causal inference studies based on observational data. Theoretical arguments and simulation results have shown that in terms of minimizing the mean squared error of an estimated treatment effect (adjusted for confounding bias through PS-based regression, subclassification, or matching), the optimal PS model is one that contains all treatment-outcome confounders, as well as all pretreatment covariates that directly affect only the outcome. Researchers have thus suggested PS variable selection procedures that explicitly use observed outcomes to identify potential confounders and prognostic variables. We show that several of these outcome-based techniques bias treatment effect estimates and erode power if naively implemented on the full data set being analyzed. We propose instead a split-data or cross-validation approach that offers a compromise between reusing versus completely ignoring outcome information when estimating propensity scores. Our method is particularly relevant in the case of high-dimensional baseline covariates, where it is infeasible to encompass all available covariates in the PS model.