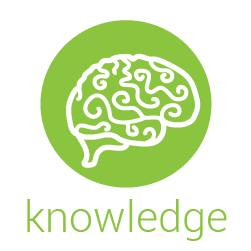
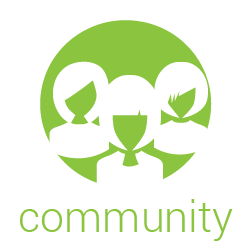
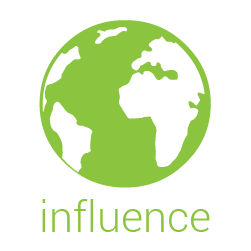
Salon 2
Developing data-light proxies for reference crop evapotranspiration estimates: A Sri Lankan case study (303245)
*Thushara Gunda, Vanderbilt UniversityGeorge M Hornberger, Vanderbilt University
Keywords: machine learning, evapotranspiration, hydrology
Similar to other agrarian nations, crop production is one of the largest consumers of water in Sri Lanka. Therefore, understanding evapotranspiration (ET) processes, which can influence up to 60% of the rainwater on land, are extremely important for effective management of agricultural water resources. To date, ET studies in Sri Lanka have been limited and provide little insight into historical ET processes in part due to large data requirements of the standard reference crop ET model of Penman-Monteith (PM). Traditionally, correction factors have been developed for less physically-based ET models such as the Thornthwaite (Th) method so that their estimates are more comparable to those of the PM. However, this approach requires that Th be first constructed with raw data prior to being adjusted. In this study, we assess whether a machine learning approach can yield comparable PM estimates to the traditional, correction factor approach without the intermediate step. This analysis is conducted with station-level data in Sri Lanka for a period of 20 years, 1991-2010. We conclude with a discussion on scaling this approach for estimating PM values with more widely available datasets.