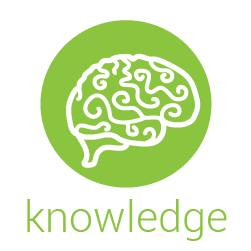
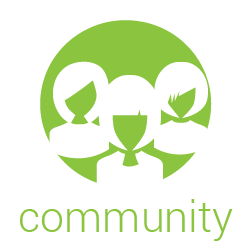
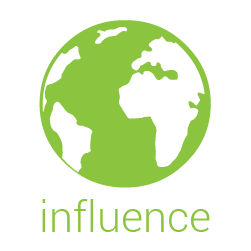
Salon 2
An Adaptive Mechanism for Multiple Queries in Differentially Private Data Synthesis (303222)
*Evercita Cuevas Eugenio, University of Notre DameFang Liu, University of Notre Dame
Keywords: differential privacy, adaptive mechanism, statistical disclosure limitation, data synthesis, multiplicative weights
Intruders may be able to identify subjects in a dataset by exploiting known connections and combining their knowledge with public information. So, when managing datasets that contain sensitive information, it is very important to properly balance protecting the privacy of individuals who contribute to databases and releasing datasets of good utility. Techniques, such as the Laplace or exponential mechanisms, are used to independently add noise to specified queries, which in turn are used to develop synthetic datasets with acceptable utility and differential privacy ensured. The model based differentially private data synthesis method assumes all queries are independently sanitized. This, however, can lead to too much noise being added to the released results because the correlation between queries is not accounted for. An adaptive mechanism that uses multiplicative weights to create a distribution that is similar to the true data, based on a specified set of queries will be presented. This adaptive mechanism considers the relationships between queries to preserve as much of the original information as possible when releasing synthetic data while still ensuring differential privacy.