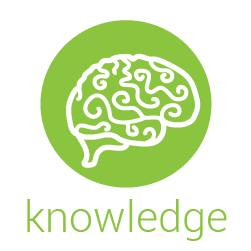
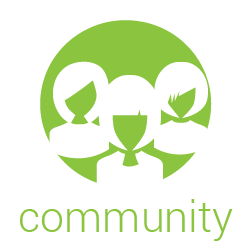
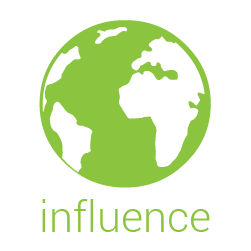
Salon 2
Kernel Fusion-Refinement for Semi-supervised Nonlinear Dimension Reduction (303217)
*Sherry Xuelei Ni, Kennesaw State UniversityKeywords: kernel fusion-refinement, dimension reduction, semi-supervised learning
In this work, a novel kernel fusion-refinement procedure with the idea of ‘minimal loss of information’ is proposed for the semi-supervised nonlinear dimension reduction problem. Numerical experiments are conducted in the framework of high-dimensional semi-supervised learning based on some popular data sets. The classification accuracy rate is used as the performance metric to quantitatively assess the proposed algorithm. The results demonstrate that the new method (named SemKFR) can efficiently handle the nonlinear features in these data sets. Moreover, the comparison between SemKFR and other algorithms also justify its competitiveness in the semi-supervised learning area.