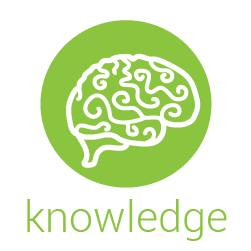
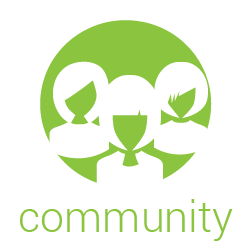
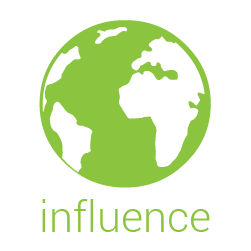
Salon 2
Kalman Filter and Interacting Multiple Model for Time Series Prediction (303198)
*Yitong Zhou, Xi'an Jiaotong-Liverpool University, Boston UniversityKeywords: Kalman Filter, Interacting Multiple Model,state-space model, stochastic process, time series, design of experiment, nonparametric test (Friedman test, Multiple Comparisons with the Best), AR model, Monte Carlo simulations
In this paper, a unified framework is utilized for online predictions based on the Kalman filter (KF). The KF has been extensively used in literature for target tracking and state estimation. In our case, it is used for forecasting non-stationary time series whose properties change over time. By embedding the Autoregressive (AR) model into the KF, we shall use it to predict real and simulated data. Furthermore, implemented as an adaptive system, a series of KF with different properties is run in parallel. This set up is called the Interacting Multiple Model (IMM), a dynamic multiple model approach which allows for switching between different models.
In addition, Design of Experiment (DOE) is also undertaken to study the influence of all factors on the performance of the filters. The optimal settings of these factors could lead to a better model specification for the KF/IMM. With the designed models already in place, nonparametric tests are carried out to assess the ranked performance of different models. Finally, we demonstrate the superiority of the KF/IMM over the classical AR model.