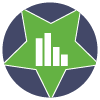
Hibiscus B
Optimizing Test Data for Longitudinal Studies (303134)
*Catherine Elizabeth Billington, WestatLaura Branden, Westat
Keywords: test data management, data quality, longitudinal studies
Creating data that can be used to test CAPI programming and systems integration before data collection starts is a critical step for data quality. Approaches to generating test data for longitudinal studies include creating custom data each round, using production data, or maintaining a test database. Many studies opt for a hybrid approach. Test requirements for multi-mode longitudinal studies also include all systems integration testing.
A case study demonstrates how CAPI test data are created, maintained, and used on one longitudinal study, including for systems integration testing. The National Health and Aging Trends Study (NHATS) gathers information in-person from a nationally representative sample of Medicare beneficiaries ages 65 and older. Annual re-interviews document change over time. The test data set parallels production data and is maintained for end-to-end testing each round. Test cases from the previous round are aged to anticipate testing needs for the upcoming round. Detailed scenario testing assesses any complex programming and specifications changes. Data are available well in advance of the field period to check preload files and all instruments. End-to-end testing assesses all systems including electronic records of calls and other paradata collected on laptops or mobile devices. This provides timely test data that are current, realistic, and reliable, and allows project staff to sustain an annual production schedule.
Maintaining test data increases the quality of collected data in a longitudinal multi-mode study and supports a compressed data collection schedule. It is costly to fix errors in the field or through editing. The effort associated with maintaining longitudinal test data and full systems integration testing is significant. However, for a complex longitudinal study, this approach represents both cost and time savings round-to-round while providing data of the highest quality.