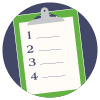
Orchid AB
Rank Question Designs and Analysis Methods (303110)
*Chia-Ling Kuo, University of Connecticut Health CenterJessica R Hoag, University of Connecticut Health Center
Keywords: Likert Scale, Ranking Scale, Simulation Study, Questionnaire Design, Surveys, Visual Analogue Scale, Quality Improvement
Surveys/questionnaires are important to identify the most important barriers physicians face to improve patient care. Typically, potential barriers (factors) are preselected by investigators and described in a question for participants to rank from most to least important. Problems may arise if not every factor applies or if multiple factors are equally ranked. Motivation for the present study originated from the survey question posed to members of the American Orthopaedic Society regarding rates of care for underinsured athletes and factors influencing the provision of care. Results suggested many participating members only ranked what they considered to be the most significant, or "top," barriers and assigned the same rank to multiple barriers. Nonsignificant, or "bottom," barriers were ranked infrequently and therefore comprised a small sample size due to many missing values. Participants also had unequal contributions to top ranks, with greater contribution from members who gave a top rank to multiple barriers. The nature of this type of ranked data can complicate data analysis, and the number of primary factors ultimately selected is generally performed ad hoc. To overcome this issue, the question can be broken down into barrier-specific questions in Likert-style or visual analogue scales (VAS). These two scales are common in psychometric research, but the choice of appropriate statistical methods remains controversial based on the nature and scale of the data. In this paper, we compare three question designs (ranking, Likert scale, and VAS) via simulations to identify primary factors. We focus our investigation on the differences in designs by the scale of data. We introduce a simulation-based method that accounts for correlation between ranks and ratings within subjects.