All Times EDT
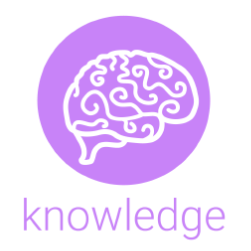
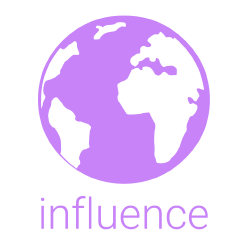
Virtual
Assessing the Precision of a Polygon Approximation Methodology in Statistical Shape Analysis with Regard to a Classification Problem (310013)
*Chalani Prematilake, East Carolina UniversityKeywords: Statistical shape analysis, statistics in manifolds, planar contours
High-dimensional data arises in a variety of fields due to technological advancements. As a result, statistical methods of analyzing high-dimensional data have become very important. But thinking of the computational cost and the time needed to execute a task, it is also important to study dimension reduction techniques. Statistical shape analysis is a field that often deals with high-dimensional data where data analysis is time-consuming. Therefore we think dimension reduction is important.
Prematilake and Ellingson (2017) discussed two different parameterizations that can be used in reducing the number of sampling points of approximating shapes of planar contours. The two parameterizations discussed are arc length parametrization, which gives equally spaced sampling points that approximate the shape, and curvature-based parameterization, which chooses points such that total curvature between each consecutive pair of sampling points is equal. Two different decision criteria were utilized under each parameterization to select a lower bound for a number of sampling points at an error threshold of 0.005. In this study, we discuss the precision of the methodology with regard to a classification problem. Furthermore, we approximate the smallest percent number of vertices that a polygon should retain in order to keep the classification without failing.