All Times EDT
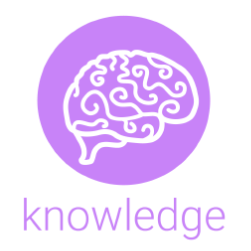
Virtual
Data-Driven Approach to Detect and Predict the Initiation of the Madden-Julian Oscillation (310004)
*Wen-wen Tung, Purdue UniversityKeywords: climate, environment, dynamical systems, machine learning, deep learning
Detecting dynamical changes in complex systems is one of the most important problems in physical science and engineering. In this work, I combine dynamical system and machine learning methods to detect the initiation of the "Madden-Julian oscillation" (MJO), a dominant mode of tropical atmospheric variability on intraseasonal (20--90-day) time scales and planetary spatial scales. In theory, the MJO is a planetary-scale nonlinear oscillator interacting with smaller-scale stochastic effects. Thus, I create statistical learning models for detecting the local changes of atmospheric states that precede MJO convection initiation, as indicated by the Real-time Multivariate MJO (RMM) index. I incorporate the spatiotemporal information of perturbation kinetic energy, water vapor, and temperature, as well as their permutation entropy (a measure of the irregularity of a complex non-stationary temporal process), in global gridded re-analysis data from 1980 to 2017. These factors are used to identify and predict the states and changes that occur as a nascent MJO emerges. Outcomes from classical statistical learning models to deep learning models will be analyzed, compared, and discussed.