All Times EDT
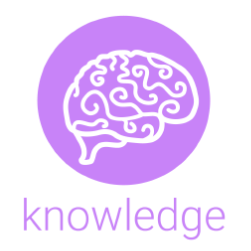
Virtual
An Empirical Bayes Approach to Estimating Dynamic Models of Co-Regulated Gene Expression (309914)
*Sara Venkatraman, Cornell UniversityKeywords: Bayesian statistics, differential equations, dimensionality reduction, omics/genetics studies
Time-course gene expression data provides insight into the dynamics of complex biological processes. It is of interest to identify genes with similar temporal expressions because such genes are often biologically related. This task is complicated by the high dimensionality of genetic datasets and the nonlinear dynamics of gene expression. We propose an empirical Bayes approach to estimating ordinary differential equation (ODE) models of gene expression, from which we derive metrics that capture similarities in two genes’ time dynamics. These metrics can be used to construct clusters or networks of functionally-related genes. Our method leverages biological databases that document known interactions between genes by using this information to define informative prior distributions on the ODE model's parameters. We then derive data-driven shrinkage parameters that optimally balance the ODE model's fit to both the data and external biological information. Using real gene expression data, we demonstrate that our biologically-informed similarity metrics allow us to recover sparse, interpretable gene networks. These networks reveal new findings about the dynamics of biological systems.