All Times EDT
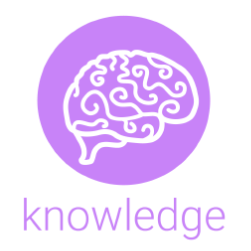
Virtual
Nonparametric Sensitivity Analysis in the Generalization of Experimental Results (309888)
*Melody Huang, University of California, Los AngelesKeywords: causal inference, sensitivity analysis, IPW, weighted estimators, generalizability, external validity
Randomized control trials (RCT) allow researchers to estimate causal effects in an experimental sample with minimal identifying assumptions. However, to generalize a causal effect from an RCT to a target population, researchers must adjust for a set of treatment effect moderators. In practice, it is impossible to know whether the set of moderators has been properly accounted for. In the following paper, I propose a two-parameter sensitivity analysis for the generalization of experimental results, with several advantages over existing methods. First, the framework does not require any parametric assumption on either the selection or treatment effect heterogeneity mechanism. Second, I show that the sensitivity parameters are guaranteed to be bounded and propose (1) a diagnostic for researchers to determine how robust a point estimate is to killer confounders, and (2) an adjusted calibration approach for researchers to accurately benchmark the parameters using existing data. Finally, I demonstrate that the proposed framework can be extended to doubly robust, augmented weighted estimators. The sensitivity analysis framework is applied to a set of Jobs Training Program experiments.