All Times EDT
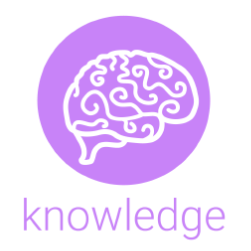
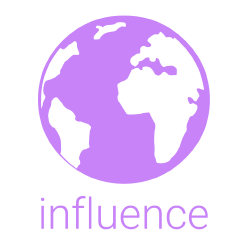
Virtual
An Analysis of Estimators in Simulation Optimization-Based Feature Selection (309878)
Sara Shashaani, North Carolina State University*Kimia Vahdat, North Carolina State University
Keywords: Feature Selection, Simulation Optimization, Robust Statistics
Finding the most informative and contributing variables for a prediction rule in big datasets is a challenging task. There are two main obstacles to this problem. First, the decision space is binary with high dimensions, and it is impossible to do an exhaustive search. Second, prediction rules often suffer from the overfitting problem which can misguide the search. Most of the current variable selection methods follow greedy algorithms that end up with sub-optimal solutions. We propose a simulation optimization-based feature selection algorithm with a focus on improving the candidate solution estimation in the simulation process as we separate the estimation and optimization steps. Our proposed estimators employ sampling schemes and influence functions that ensure low bias and variance. We show improvement in several simulated and real datasets in comparison with the state-of-the-art algorithms.