All Times EDT
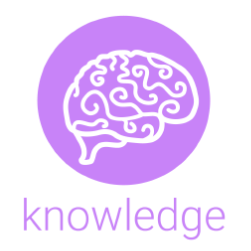
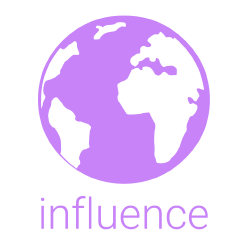
Virtual
The Interplay of Demographic Variables and Social Distancing Scores in the Deep Prediction of US COVID-19 Cases (309868)
*Francesca Tang, Princeton UniversityKeywords: Community Detection, COVID-19, Machine Learning, Neural Networks, Spectral Clustering
We introduce a novel approach to capture implied volatility smiles. Given any parametric option pricing model used to fit a smile, we train a deep feedforward neural network on the model's orthogonal residuals to correct for potential mispricings. Using a large number of recent S\&P500 options, we compare our hybrid machine-corrected model to several standalone parametric models ranging from ad-hoc corrections of Black-Scholes to more structural no-arbitrage stochastic volatility models. Empirical results based on out-of-sample fitting errors - in cross-sectional and time-series dimensions - consistently demonstrate that a machine can in fact correct existing models, without overfitting. Moreover, we find that our two-step technique is relatively indiscriminate: regardless of the bias or structure of the original parametric models, our hybrid approach is able to correct them to approximately the same degree. Hence, our methodology is adaptable and versatile in its application to a large range of parametric option pricing models. In addition, machine corrected methods outperform purely machine learning methods, without using an option pricing formula as a starting point.