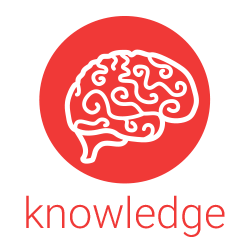
Evergreen Ballroom H
Real-Time Prediction of Bitcoin Bubbles and Crashes (306571)
*Min Shu, Stony Brook UniversityWei Zhu, Stony Brook University
Keywords: Cryptocurrency, Bitcoin, Bitcoin bubble, Bitcoin Price, Bubble prediction, Log-periodic power law singularity, LPPLS, Market crash
In the past decade, Bitcoin has become an emerging asset class well known to most people because of their extraordinary return potential in phases of extreme price growth and their unpredictable massive crashes. Due to the sharp change and large volatility of Bitcoin price, the conventional financial bubble detecting methods do not provide an effective identification of Bitcoin bubbles. We propose the adaptive multilevel time series detection methodology based on the Log Periodic Power Law Singularity (LPPLS) model to effectively detect bubble. Our analysis shows that the LPPLS confidence indicator based on this new method is an outstanding instrument in effectively detecting the bubbles and accurately forecasting the bubble crashes, even if a bubble exists in a short time. The short-term indicator provides useful insights into the bubble status on daily time scale, while the long-term indicator on weekly scale. The novel method can provide real-time detection of bubbles as well as timely information to warn of an imminent crash for not only the cryptocurrency market but also other financial markets.