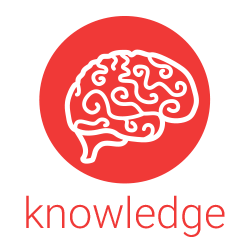
Evergreen G
Revisiting the Gelman-Rubin Diagnostic (306447)
Dootika Vats, Indian Institute of Technology Kanpur*Christina Phan Knudson, University of St. Thomas
Keywords: markov chain monte carlo, bayesian, convergence,
Gelman and Rubin's (1992) convergence diagnostic is one of the most popular methods for terminating a Markov chain Monte Carlo (MCMC) sampler. Since the seminal paper, researchers have developed sophisticated methods of variance estimation for Monte Carlo averages. We show that this class of estimators finds immediate use in the Gelman-Rubin statistic, a connection not established in the literature before. We incorporate these estimators to upgrade both the univariate and multivariate Gelman-Rubin statistics, leading to increased stability in MCMC termination time. An immediate advantage is that our new Gelman-Rubin statistic can be calculated for a single chain. In addition, we establish a relationship between the Gelman-Rubin statistic and effective sample size. Leveraging this relationship, we develop a principled cutoff criterion for the Gelman-Rubin statistic. Finally, we demonstrate the utility of our improved diagnostic via an example using Titanic survival data: Jack and Rose's probabilities of surviving are markedly more precise using our updated Gelman-Rubin statistic.