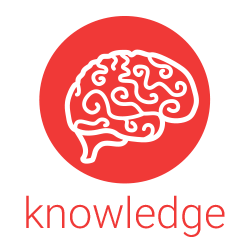
Evergreen A
Bayesian Hierarchical Factor Regression Models to Infer Cause of Death from Verbal Autopsy Data (306406)
David Dunson, Duke UniversityAmy H. Herring, Duke University
*Kelly R. Moran, Duke University
Elizabeth L. Turner, Duke University
Keywords: Bayesian, Cause of death, Covariance regression, Factor analysis, Forensic data, Semi-supervised classification, Verbal autopsy
In developing countries it is often of critical interest to determine and study the cause of death (COD) for individuals and the cause-specific mortality fraction (CSMF) for populations. Post-mortem autopsies, considered the gold standard for COD assignment, are generally not performed when individuals die outside the hospital. In many settings, even when individuals die in the hospital, full autopsies are difficult or impossible to implement due to expense and/or cultural norms. Thus data on COD is often scarce or unrepresentative of the entire population of deaths, and the CSMF is often poorly estimated. For this reason, Verbal Autopsies (VAs) are commonly conducted, consisting of a questionnaire recording demographic information, known medical conditions, symptoms, and other factors for the recently deceased. VA data consist of multivariate mixed scale observations for each individual. COD may be directly measured for a subset of labeled individuals, or labeled COD data may come from an external data set. The goal of this article is to improve modeling of VA data to infer more accurately COD and CSMF, while providing accurate uncertainty quantification in associated inferences.