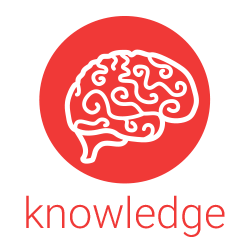
Evergreen A
Withdrawn-Heterogeneity-Adjusted Test Statistics (306387)
*Luella Fu, San Francisco State UniversityBowen Gang, University of Southern California
Gareth James, University of Southern California
Wenguang Sun, University of Southern California
Keywords: empirical Bayes, multiple testing, unequal variances
Multiple testing frameworks are routinely employed by scientists and researchers to compare many features simultaneously across many groups. For example, biologists often compare gene expression levels across treated and untreated groups in order to find genes that respond to treatment. Generally, multiple testing involves three steps: reduce the data to standardized test statistics, usually z-values; calculate p-values or analogous statistics based on z-values; and finally find a threshold of significance that corrects for multiplicity. The standardization step is upheld as conventional wisdom, but this research demonstrates through theory and intuitive examples that standardization has negative effects for heteroscedastic multiple testing. We propose an ameliorating testing procedure, the“heterogeneity adjusted test statistic” (HATS) procedure, suitable for large-scale, heterogeneous data. It eliminates the need to standardize heteroscedastic errors and provides an optimal multiple testing procedure for finding non-zero means.