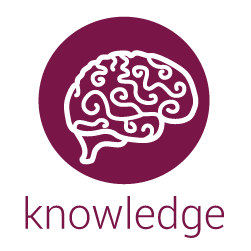
Salons FG
What Machine Learning Can and Cannot Do (304833)
*Claudia Perlich, Two SigmaKeywords: Machine Learning, Statistics, Data Science, Modeling
Predictive Modeling and Supervised Learning are staple techniques in the Data Science arsenal of algorithms. The origins of some of those solutions trace back more than 50 years, but with the recent explosion of data and the wide adoption of data technologies they are receiving a new level of attention. This talk takes on some of the more broadly asked question around predictive modeling and machine learning: Where do we stand today? What can AI and machine learning really do well? On the flip side: When does it even make sense to try predictive modeling? What are the key success factors of making prediction work? And when do predictive models fail? The talk will touch on those questions and cover a wide number of case studies where skillful adjustments and alternative perspectives provide creative solutions.