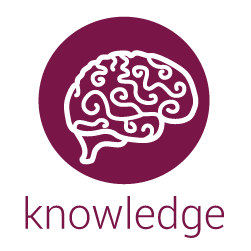
Salons HI
Causal Inference for Infectious Disease Interventions in Networks (304775)
*Xiaoxuan Cai, Yale UniversityForrest Crawford, Yale University
Keywords: Causal inference, Infectious disease, Survival analysis
Measuring the effect of infectious disease interventions (vaccinations) is a major challenge in contemporary epidemiology, because the outcome of interest - infection - is transmissible between individuals. This complication means that individuals' infection outcomes may depend on the treatments and outcomes of other individuals, a phenomenon known as "interference" or "spillover". Infectious disease interventions are unique because they can have distinct effects on individual-level susceptibility to disease, and infectiousness once infected.
In our work, we propose a general stochastic model of infectious disease transmission in continuous time, and natural definitions of causal estimands for individual-level direct and indirect vaccine effects. This framework provides the methodological foundations for practical causal inference in interconnected study populations. We develop semi-parametric statistical models and an inferential procedure for estimating vaccine effects, which involve multiple time scales and permit regression adjustment for variables associated with susceptibility to disease, and infectiousness once infected.