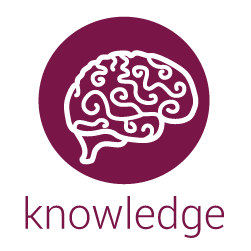
Rosewood
Data Science for Development and Justice (304908)
*Anjali Mazumder, Carnegie Mellon UniversityData is everywhere and being collected continuously: People are using mobile phones to take photos, send texts and record steps taken. Organizations collect data from incident reports, real-time service delivery tracking, satellite images and surveys. Almost every modern organization, be it for-profit, non-profit or government is inundated with data derived by digital collection of information (credit card swipes, remote sensors, CT scans, on-line transactions, wearables, etc.). Documents (historic and current), tweets and other messaging forms provide data in the form of text, unearthing new ways for historians, political scientists, sociologists, etc. to generate insights from such information. Scientists, business analysts, and decision-makers alike wish to harness data to inform decision-making in policy and practice. However, it is not always clear how best to use and harness the data in actionable way for decision-making and reasoning (under uncertainty) for policy or practice. Further, as data and infographics are splashed on newspapers and on the internet, the public is becoming informed about important societal issues in unprecedented ways and there is a growing interest and understanding in how data can change the way we live and improve services to better our lives and communities.
Constant supply of data produced by companies, think tanks, government agencies, independent researchers, academics and others is a significant and rich resource. Rapid development of large-scale data collection technology has ignited research in spatial-temporal methods, latent variable models, (social) network theory, and classification/clustering algorithms, etc. A common task in analysing modern high-throughput technologies is to try to detect relationships of dependence between variables and how these dependences change over time, accounting for causal mechanisms such as unobserved confounders or change-point events that may affect outcomes and where experimental data may be difficult or unethical to collect. Revealed dependence structures or unobserved latent patterns are often a challenging task in practice but can be used to guide data collection or dimensionality reduction and inform complex decision-making, exploiting graph modular tools. Effective use of exploratory data analysis can also provide insights and deflate the misuse and misunderstanding of fundamental numeracy and statistical concepts which can confuse, distract and derail public understanding.
With a surge in the field of Data Science, the impact and potential of effectively interrogating, distilling and evaluating big and or disparate sources of data is now widely recognized, offering important and long-lasting benefits to society. Skills to effectively interrogate, analyze and generate insights from such diverse, large and data rich resources whist formulating clear research policy questions based on an understanding the domain knowledge has become a dying art. This talk will focus on current initiatives at Carnegie Mellon University to harness insight from both low stakes (publicly available) and higher stakes (privacy or client driven) data that provides both statistical and policy insights in areas of development and justice.