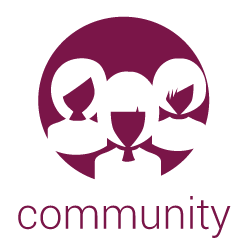
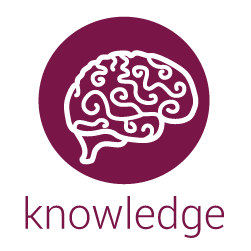
Rosewood
Missing Imputation of Cancer Proteome with Iterative Prediction Model (304786)
*Shrabanti Chowdhury, Icahn School of Medicine at Mount SinaiWeiping Ma, Icahn School of Medicine at Mount Sinai
Pei Wang, Icahn School of Medicine at Mount Sinai
Lin Chen, The University of Chicago
Keywords: Missing; Imputation; Cancer; Proteomics; EM
Due to the dynamic nature of mass spectrometry (MS) instruments, data from MS based proteomics experiments often contains a large number of missing values imposing a great challenge to proteomics data analyses. The missing events in MS based proteomics data are not missing at random and, more specifically missing probability is highly correlated with protein abundances. We propose a novel imputation method specifically designed for proteomics data-ADMIN (Abundance dependent missing imputation) by using the correlation structure among the highly correlated (similar abundance profiles) proteins, modeling the abundance dependent missing pattern through an EM-based algorithm. To evaluate the performance of ADMIN, we developed a simulation framework by generating pseudo datasets from CPTAC (Clinical Proteomic Tumor Analysis Consortium) cancer studies. For performance evaluation on the real data, we used technique replicates of the same set of patients from a CPTAC ovarian study. We considered normalized root-mean-square deviations and correlation coefficients as metrics of evaluation. ADMIN is compared with commonly used algorithms: softImpute, KNN-based imputation, and missForest.