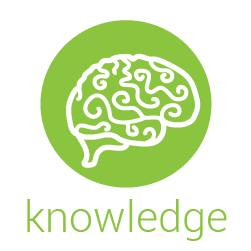
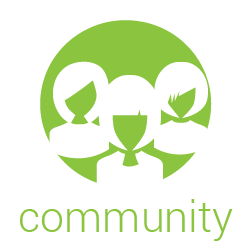
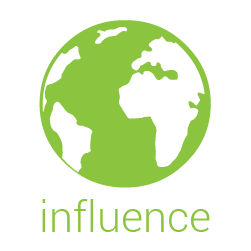
Salon 2
Variable selection methods for identifying predictor and predictor interactions associated with repeatedly measured binary outcomes (303238)
Yunyun Jiang, Medical University of South Carolina*Bethany Lynn Wolf, Medical University of South Carolina
Keywords: predictor interactions, variable selection, glmmLasso, GMMBoost, two-step algorithm
Predicting patient disease risk over time may require modeling interactions among variables and within subject correlation. Generalized linear mixed models (GLMMs) can model interactions and within subject correlation, but interactions should be specified a priori and sufficient data are needed to model interactions and main effects. Variables can be selected using stepwise selection, but these methods produce unstable estimates. GlmmLasso, a GLMM adaptation of lasso regression, is an alternative to stepwise selection that penalizes the likelihood to yield sparse models. GMMBoost, another variable selection algorithm, yields sparse models through iterative reweighting of residuals. Both glmmLasso and GMMBoost have been shown to produce biased estimates. We propose a 2-stage approach to address the bias in estimates from glmmLasso and GMMBoost. Simulation studies show that glmmLasso and GMMBoost more effectively recover variables and interactions associated with a repeated binary outcome than step-wise selection. We also show the 2-stage approach for glmmLasso and GMMBoost reduces parameter estimate bias. We apply each method to 2 clinical datasets and compare the resulting models.